4 lessons from Kafka Summit London 2024
What we learned, from shifting left with governance to getting data integration right.
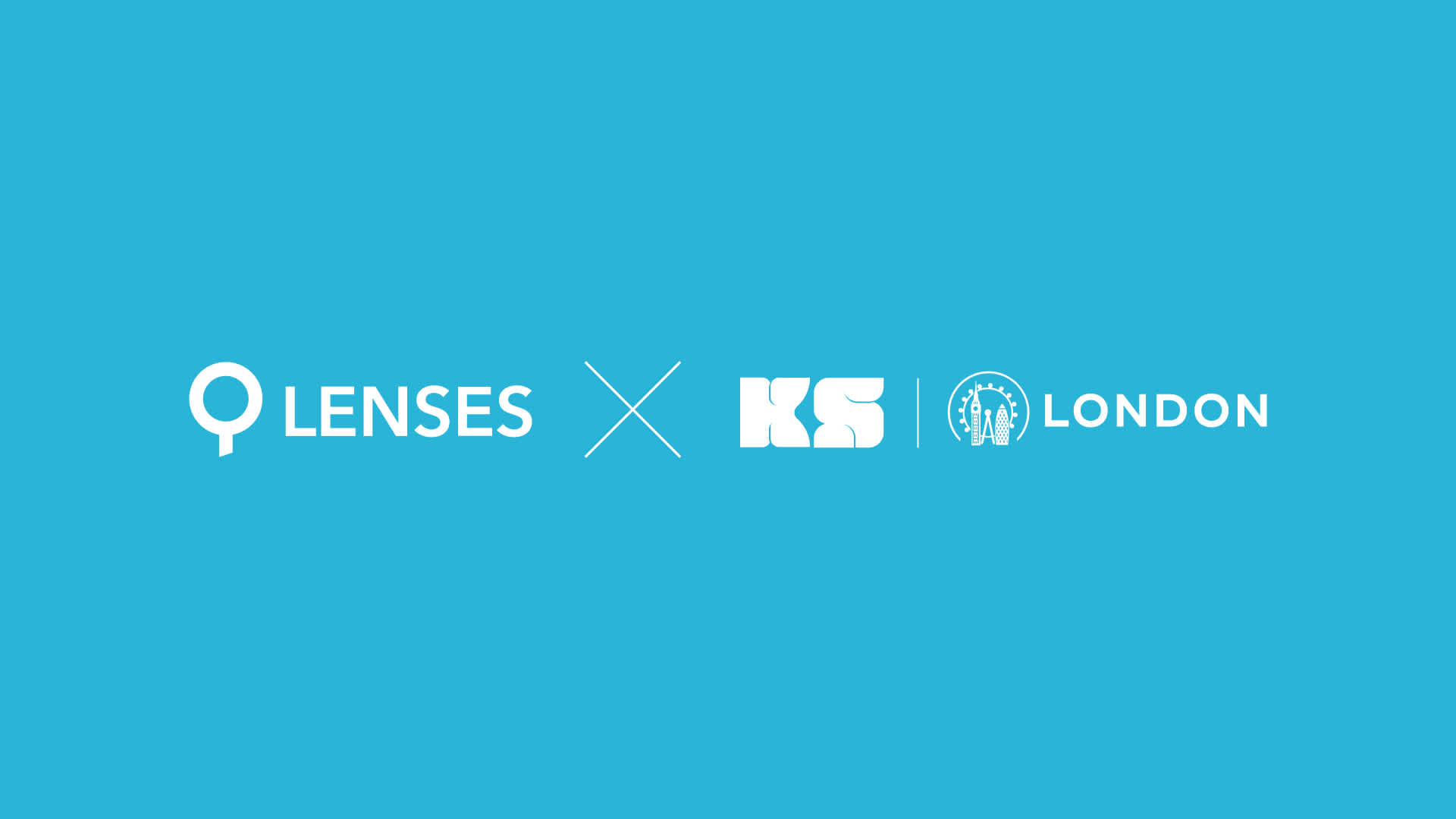
It was lovely to see so many of the community and hear about the latest data streaming initiatives at Kafka Summit this year. We always try to distill the sea of content from the industry’s premier event into a digestible blog post.
This time we’ll do it slightly differently and summarize some broader learnings, not only from the sessions we saw, but the conversations we had across the two days.
This year at Kafka Summit, governance took center stage. It was announced during the keynote that Confluent has beefed up its streaming governance offering by making it available by default.
But the story told by Jay Kreps, Shaun Clowes and team also spoke to a more general market trend. Firstly, teams need to shift left and give developers more autonomy to manage streaming data, thereby increasing productivity and innovation. Second, that engineering teams are rearchitecting a huge number of applications to be streaming-native.
Both these points mean that more developers need access to Kafka to be productive. More developers accessing Kafka means data needs to be discoverable and understandable, and there should be guardrails for how products can be built as safely and efficiently as possible.
Whether Kafka Connect or Apache Flink, data integration was the talk of the summit this year. The number of new data sources being onboarded by organizations only continues to snowball, fueled by AI projects.
It was good to hear the community expanding the ways these data pipelines can be integrated – from building Kafka Connectors with Kotlin to decoding the data integration Matrix.
It was also an opportunity to celebrate the democratization of Kafka Connect Connectors.
CERN discusses how they decode data from IoT devices connected to a LoRa network using Apache Kafka, Kafka Connect and Kafka Streams. Their data streaming service is made up of NILE components (Internetworking Laboratory Environment) and complemented like so:
They connected to their IoT devices, optimizing for cost and covering 50 gateways providing coverage to the offices and campus. Devices are deployed in different places, connected to the LoRa gateway, which are then integrated with the cable network using MQTT:
We were proud to see CERN’s usage of Lenses Open-Source Kafka connectors in their IoT pipelines.
We’re committed to providing the community with a choice of high-quality OS connectors that can be used in critical projects like CERN’s. Many of you helped us shape our roadmap. Your favorite connectors include Azure Event Hubs (out now), Apache Iceberg, and Kafka-to-Kafka replication, which you upvoted at Kafka Summit:
We are about to revolutionize the way we live and work. This talk by the CEO of Airy, Steffen Hoellinger, was a portal into the near future. He demonstrated how intelligent custom chatbot UIs can be integrated with data in the stream to drive better business automation and experiences.
Steffen says that AI will not make us 10x or 100x more productive at work, but 10,000x. Yet only when models are trained on data in the stream for freshness. Looking ahead, AI co-workers will not only assist us, but make certain decisions on our behalf.
Imagine asking your AI co-worker a question: “What meetings do I have today?” Not only does it check through the latest emails, messages and calendar updates to share your agenda, but it recommends re-prioritizing or rescheduling meetings based on childcare cancellations, traffic jams, and critical business incidents that came up seconds ago.
Not only will we have generative AI co-workers, but consumer-facing assistants are also on the way. AI-powered capabilities for shoppers could become our hyper-personal shoppers, knowing exactly what to order for a weekly grocery shop based on what’s missing from the fridge.
Every application associated with an AI-driven shopping experience will require real-time data to provide a continuous flow of information. Intelligent co-workers and personal assistants will sit between the foundational AI models and data streaming:
To create this critical instant feedback loop and quality user experience, the pressure is on for technologists to integrate AI models with organizational data, live in the stream. The pressure is high, as the quality of AI output directly influences the relevance of the data and the decisions taken.
This is how we see the next year going for data engineering, where real-time AI applications will gain momentum with market leaders, from boosting employee productivity to disrupting entire consumer markets.
When Kafka was created in 2011 at LinkedIn, it was a different world. Most companies were still on-prem. The notion of cloud computing was just beginning to emerge.
The success of Kafka means that streaming technologies are evolving.
Naturally, streaming platforms need to diversify, and need to cater for different types of organizations and requirements. Some require more focus on cost, others on latency, others on security. We see a greater shift towards streaming platforms that address different challenges and technologies, whilst respecting the same Kafka APIs.
Early-stage start-ups like WarpStream, for example, are building affordable, cloud-native data streaming services, which are more appropriate for a specific set of companies or workloads.
Another interesting streaming start-up we spoke to was Imply. From the original creators of Apache Druid, Imply is a real-time analytics platform working at high speed and scale.
These two start-ups are a testament to what Shaun Clowes shared in the Kafka Summit opening keynote about universal data products: a product where the analytical and operational come together, with data streams as the perfect substrate.
We can’t wait to see more of this at Current in September.
_____
Want to continuously learn about the frontiers of Kafka and beyond? Join our weekly Kafka Live Stream.